“Dubrovnik Ghost Series” / Neural Networks as a Diffractive Medium (Part 2)
This post is the second half of my final report for Computational Arts Based Theory and Research, 2019. It follows on from Part 1 which you can find here.
Data providence, diffraction and “personal” latent space
My father John Bayliss was, in his spare time, a landscape painter. He taught me to draw and paint at a young age and some of my favourite memories of time spent with him were out in the Australian bush, hot in the shade, annoyed by blowflies, drawing and painting the latest river, tree, or dusty path moving up to the horizon.
Time – years; and distance. I move to the UK. Before he passed away, my father and I regularly discussed having one more painting trip together but logistics got in the way. His health deteriorated, and the closest we got to having that trip together was a short trip down to the local lake in Canberra, near the hospital, for a bit of sketching in the car.
After he passed away, I was tasked with putting together a film for the funeral, and as part of that process I archived many of his paintings, most completed before I was born. I’ve had these archives since and it was only whilst researching for this project I realised I might be able to revisit them and possibly create a new experience shared with my memory of him.
–
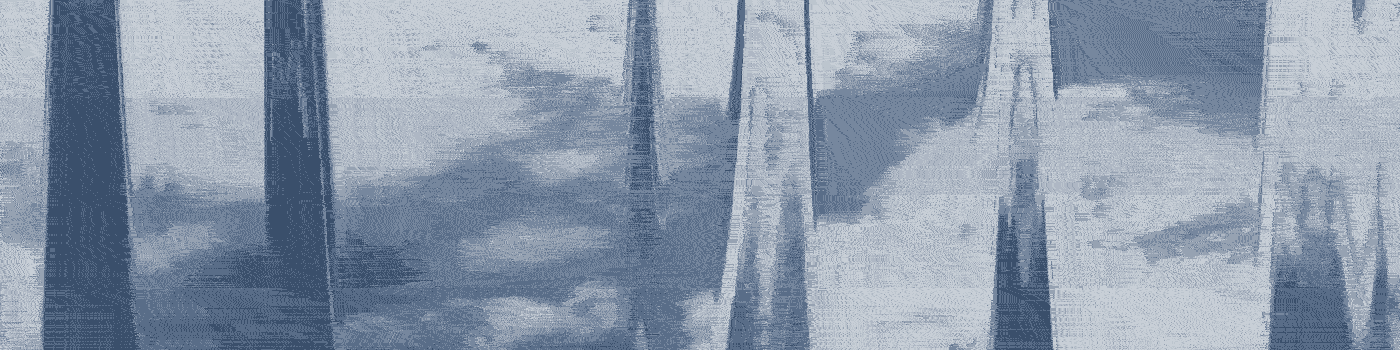
In her essay “Diffracting Diffraction: Cutting Together-Apart ” Karen Barad (2014) lucidly establishes an ontological framework drawn from quantum physics and employs it to examine notions of identity, gender, entanglement and materiality.
At the core of this idea is the concept of diffraction, a term initially used in physics to explain the spreading of waves around obstacles. This term has been repurposed by Haraway (1992) and later and expanded upon by Barad (2014, p.p 2), who states:
Diffraction is not a set pattern, but rather an iterative (re)configuring of patterns of differentiating-entangling. As such, there is no moving beyond, no leaving the ‘old’ behind. There is no absolute boundary between here-now and there-then. There is nothing that is new; there is nothing that is not new.
Looked through this diffractive lens, it is clear how a neural network may provide unique access to a formulation of entangled spacetimematterings. Each data point in the dataset represents a distinct there/then; once analysed by the network it becomes a reference point in a high dimensional latent space – a locus within a hyper-landscape. Through the intra-action between these disparate datapoints and the new conditional data (in the form of new paintings and sketches provided by me in the here/now), new “scenes of entanglement” (Blackman, 2019) may emerge.
When the data used within a neural network, GAN in this case, has a unique story or personal providence, the network (in collaboration with the artist) may, in the words of Lisa Blackman, “attempt to re-move and keep alive what becomes submerged or hidden” (2019, p. p 94).
–
Come down to us
As a way of highlighting what the medium affords it might be valuable to contrast it to other artists working with memory and personal archives to situate GAN and neural network practices more precisely.
Morehshin Allahyari incorporates personal memories and archives into much of her work. She coined the term Re-Figuring in relation to her work entitled “She who sees the unknown” (2018) as a feminist and activism practice. She asks “How can we re-imagine an other kind of present or future through re-imagining the past”. In her earlier work “The Recitation of A Soliloquy” (2012), Allahyari found a page from her mother’s diary which was written whilst her mother was pregnant with Allahyari. The artist then inscribed the words onto film, one frame at a time, to be played in a loop. These images are then edited into a film intercut with the artist’s face covered in projections of Google Maps images of Iran. The effect creates a similar diffracted spacetimemattering to that of a neural network, with the core difference being the agency behind the curation of images is entirely the artist’s.
Collaboration with a neural network requires some relinquishing of agency from the artist. Some of the most notable GAN artwork positions the neural network as a “talisman” (Gillespie, 2014), as it fits contemporary curiosity and anxieties relating to imminent automation – “the robots are coming!”
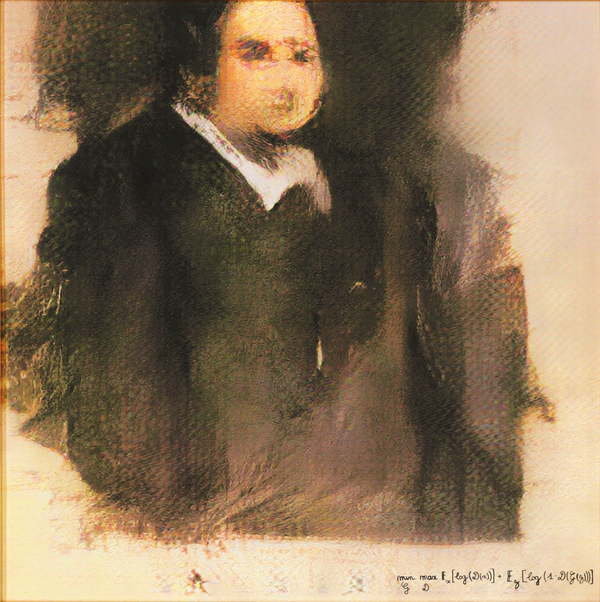
The most high profile GAN work to date, “Portrait of Edmond Belamy” by French collective “Obvious” is a clear example of how “otherness of the AI” currently defines and situates the work. Within the frame, beneath the generated portrait (which the artists themselves selected, from myriad other generated images), they position the differential equation which in theory will generate this specific image. This finishing touch of showmanship, techno-mysticism, and mythology building (many of the works by Obvious come with fictional character bios that accompany their generative portraits), is perhaps what launched this particular work into the public consciousness.
Contrast this with the developer of much of the technology actually used by Obvious to create the image, Robbie Barrat, who commented on Twitter “I feel really guilty using so much art history just as fuel for a GAN – without even looking at most of it.” Barrat is an incredible technology prodigy, a young artist, and his comment may also be read as one way to interpret a lot of GAN imagery. Incredibly sophisticated tech but it is only as interesting as the contextual mileu it arises out of, without careful reflection and curation then it is reduced to functioning as simply another software trick marking the current techno-zeitgeist from the next. Sounds “Obvious” when put like that, I must say.
James Vlahos’ “Dadbot” shares a similar origin story to the topic of my research. In 2016 his father was diagnosed with cancer and Vlahos’ response was to attempt to capture as many recollections from his father through long taped audio interviews. These interviews became the source text for a mobile “chatbot” app which Vlahos and others were able to interact with, receive small anecdotes from and receive text messages from. Like the aforementioned GAN work by Obvious the Dadbot made waves within the mainstream media. In a reflective piece written some time after the initial rush of press attention, Vlahos notes an interesting anecdote – “While most people simply expressed sympathy, some conveyed a more urgent message: They wanted their own memorializing chatbots.” His work struck a chord with a wider public. This theme of personal reflection and “digital archiving” resonates with me – neural networks don’t (yet) offer some form of posthuman vessel for infinite life. What I do think they afford is a new manner in which we may reflect on and reinterpret our experience and preconceptions from a middle distance, and in doing so gain new insights.
In “Blade Runner – Autoencoded” (2017), Terence Broad and Mick Grierson highlight the technical and aesthetic affordances of a neural network through the process of training an Autoencoder (a distinct type of image processing neural network) to “learn” how the film Blade Runner looks. Their paper elegantly explains how the technology works they also note an aesthetic attribute of neural network generated material – “Some of the flaws in its visual reconstruction are reminiscent of the deficiencies of our own, especially regarding memories of dreams.”
Through my own practice I have found that GAN generated artwork is imbued with a spectral quality not present in a straightforward recombination of observed images. As the network attempts to maximise the coherence of the new data to the existing model it can create visual artefacts, bursts of light and shadow that highlight the materiality of this new “other”.
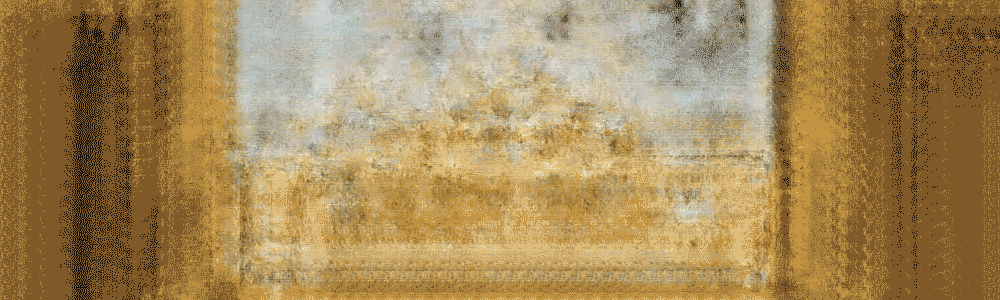
In Ghosts of My Life (2014), Mark Fisher describes the importance of “crackle” in an audio recording, “the sounds of technologies breaking down.” “.. Crackle makes us aware that we are listening to a time that is out of joint; it won’t allow us to fall into the illusion of presence.” (2014, p.p. 30) The visual “crackle” created by the GAN evokes a similar sense of un/time, of things being out of joint.
Within a GAN, time is out of joint. Diffracted. Entangled. “Entanglements are not unities. They do not erase differences; on the contrary, entanglings entail differentiatings, differentiatings entail entanglings. One move – cutting together-apart.” (Barad, p.p 10)
–
And / End
This leads, finally, to the contemporary entanglement between myself and this research practice. The “lost future,” the road trip un/taken. The actual road trip taken was to Dubrovnik in Croatia, somewhere my father was never able to visit. Dubrovnik is a beautiful walled city, surrounded by vibrant coastal landscape not too dissimilar from the places in Australia where my father and I used to paint. This turns out to be a useful similarity – the diffractive nature of the GAN highlights both what was and what wasn’t – if my father hadn’t painted any images where the mountains were green, then they wouldn’t be part of the dataset. They didn’t happen, and therefore they would not be diffracted back through the GAN when I showed it my sketch. As a result, the images may only be reconfigurations of places he’d been. In this respect, the GAN is emphasising the gulf in distance and time between my memories shared with my father, the spacetimematterings within which he first created the images subsequently used as the dataset, and the contemporaneous moments within which I create these new works with his memory.
In “And – Phenomenology of the End” (2015), Franco Berardi asks:
.. how can we be in touch with the register of time, how can we feel the flow of living matter? Memory is our access to this register of time, and as everybody knows, memory is not a regular, fixed, repeatable, computable re-enactment of an event, or a series of events. Memory is the recreation and re-imagination of a past that is continuously changing as long as we distance ourselves and our viewpoint changes. (p287 – emphasis added)
It is this aspect which perhaps best sums up my experience working with neural networks and how I now perceive them as an artistic medium. The work is best appreciated as an ongoing process, a dialog between artist and layered memory that may be diffracted through with new insights unearthed. It is an exciting and unpredictable technology and no doubt will play a large role in computational arts in the near and distant future.
–
References
- YouTube. 2019. Artificial Imperfection | MoMA R&D Salon 24 | MoMA LIVE – YouTube. [ONLINE] Available at: https://youtu.be/AnBfSwyqtdY?t=499. [Accessed 13 May 2019].
- YouTube. 2019. “And: Phenomenology of the End,” lecture by Franco “Bifo” Berardi – YouTube. [ONLINE] Available at: https://youtu.be/Cb62DKZ5qSY?t=2808. [Accessed 13 May 2019].
- The Verge. 2019. ThisPersonDoesNotExist.com uses AI to generate endless fake faces – The Verge. [ONLINE] Available at: https://www.theverge.com/tldr/2019/2/15/18226005/ai-generated-fake-people-portraits-thispersondoesnotexist-stylegan. [Accessed 13 May 2019].
- The first piece of AI-generated art to come to auction | Christie’s . 2019. [ONLINE] Available at: https://www.christies.com/features/A-collaboration-between-two-artists-one-human-one-a-machine-9332-1.aspx. [Accessed 13 May 2019].
- Blackman, L., 2019. Haunted data: affect, transmedia, weird science, London ; New York ; Oxford ; New Delhi ; Sydney: Bloomsbury Academic.
- I. Goodfellow, et al., “Generative Adversarial Nets,” Advances in Neural Information Processing Systems (2014) pp. 2672–2680.
- What is synaptic plasticity? – Queensland Brain Institute – University of Queensland. 2019.[ONLINE] Available at: https://qbi.uq.edu.au/brain-basics/brain/brain-physiology/what-synaptic-plasticity. [Accessed 13 May 2019].
- Hayles, N. K, 2005. My mother was a computer. Digital subjects and literary texts. University of Chicago Press. p.p. 2
- Brock, A., et al, “Large Scale GAN Training for High Fidelity Natural Image Synthesis,” arXiv:1809.11096
- Study takes aim at biased AI facial-recognition technology. 2019. Study takes aim at biased AI facial-recognition technology. [ONLINE] Available at: https://phys.org/news/2019-02-aim-biased-ai-facial-recognition-technology.html. [Accessed 13 May 2019].
- T Broad and M Grierson, “Autoencoding Blade Runner: Reconstructing Films with Artificial Neural Networks,” Leonardo 2017 50:4, pp. 376-383
- MIT Press Journals. 2019. MIT Press Journals. [ONLINE] Available at: https://www.mitpressjournals.org/doi/abs/10.1162/LEON_a_01455. [Accessed 13 May 2019].
- Allahyari, M. 2019. She Who Sees the Unknown (2017 – present) – Morehshin Allahyari. [ONLINE] Available at: http://www.morehshin.com/she-who-sees-the-unknown/. [Accessed 13 May 2019].
- Allahyari, M. 2012. Recitation Soliloquy. [ONLINE] Available at: http://www.morehshin.com/recitation-soliloquy/. [Accessed 13 May 2019].
- Gillespie, T. Algorithm [draft] [#digitalkeywords] – Culture Digitally. 2019. [ONLINE] Available at: http://culturedigitally.org/2014/06/algorithm-draft-digitalkeyword/. [Accessed 13 May 2019].
- Fisher, M., 2014. Ghosts Of My Life: Writings On Depression, Hauntology And Lost Futures. Zero Books.
- WIRED. 2017. A Son’s Race to Give His Dying Father Artificial Immortality | WIRED. [ONLINE] Available at: https://www.wired.com/story/a-sons-race-to-give-his-dying-father-artificial-immortality/. [Accessed 13 May 2019].
- The Verge. 2018. How three French students used borrowed code to put the first AI portrait in Christie’s – The Verge. [ONLINE] Available at: https://www.theverge.com/2018/10/23/18013190/ai-art-portrait-auction-christies-belamy-obvious-robbie-barrat-gans. [Accessed 13 May 2019].
- Bifo, F., 2015. And: Phenomenology Of The End (semiotext(e) / Foreign Agents). Semiotext(e).
- twitter.com. 2019. No page title. [ONLINE] Available at: https://twitter.com/DrBeef_/status/1098398518400606208. [Accessed 13 May 2019].
- Karen Barad (2014) Diffracting Diffraction: Cutting Together-Apart, Parallax, 20:3, 168-187, DOI: 10.1080/13534645.2014.927623
- Haraway, D. The Promises of Monsters: A Regenerative Politics for Inappropriate/d Others Lawrence Grossberg, Cary Nelson, Paula A. Treichler, eds., Cultural Studies (New York; Routledge, 1992) , pp. 295-337.
- vice. 2019. The Robots Are Coming, and They Want Your Job – VICE. [ONLINE] Available at: https://www.vice.com/en_uk/article/kz5a73/the-robots-are-coming-and-they-want-your-job. [Accessed 13 May 2019].
- leapsmag. 2019. Dadbot, Wifebot, Friendbot: The Future of Memorializing Avatars – leapsmag. [ONLINE] Available at: https://leapsmag.com/dadbot-wifebot-friendbot-the-future-of-memorializing-avatars/. [Accessed 13 May 2019].
- Ars Technica. 2019. Why Google believes machine learning is its future | Ars Technica. [ONLINE] Available at: https://arstechnica.com/gadgets/2019/05/googles-machine-learning-strategy-hardware-software-and-lots-of-data/. [Accessed 13 May 2019].